Optimisation methods for Bayesian inference
With Audrey Repetti, Heriot-Watt University
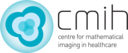
Optimisation methods for Bayesian inference: Application to high dimensional inverse problems
An important number of scientific and technological applications (ranging from healthcare to astronomy) consist in solving high dimensional inverse problems, where an unknown object is estimated from the provided measurements. A common method to solve these problems is to rely on a Bayesian maximum a posteriori (MAP) approach. A main limitation of this approach is that it does not provide any information regarding the uncertainty in the solution delivered. This analysis is particularly important in imaging problems that are ill-posed or ill-conditioned, for subsequent decision making processes (e.g. decision concerning a tumor appearing on a brain image from MRI ).
In this presentation I will present a methodology to probe the data and perform uncertainty quantification. In the proposed method, we quantify the uncertainty associated with particular structures appearing in the MAP estimate, obtained from a log-concave Bayesian model. A hypothesis test is defined, where the null hypothesis represents the non-existence of the structure of interest in the true image. To determine if this null hypothesis is rejected, we use the data and prior knowledge. Computing such test in the context of imaging problem is often intractable for state-of-the-art Markov chain Monte Carlo algorithms, due to the high dimensionality involved. In this work, we formulate the Bayesian hypothesis test as a convex minimization problem, which is subsequently solved using a proximal primal-dual algorithm. The proposed method is applied to astronomical and medical imaging.
Joint work with Marcelo Pereyra and Yves Wiaux
- Speaker: Audrey Repetti, Heriot-Watt University
- Friday 01 June 2018, 14:00–15:00
- Venue: MR11, Centre for Mathematical Sciences.
- Series: CMIH seminar series; organiser: Rachel Furner.