Mathematics and Machine Learning
Universal Adversarial Perturbations
With Alhussein Fawzi; UCLA, DeepMind
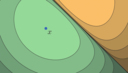
Universal Adversarial Perturbations: Fooling Deep Networks with a Single Image
The robustness of classifiers to small perturbations of the data points is a highly desirable property when the classifier is deployed in real and possibly hostile environments. Despite achieving excellent performance on recent visual benchmarks, I will show in this talk that state-of-the-art deep neural networks are highly vulnerable to universal, image-agnostic, perturbations. After demonstrating how such universal perturbations can be constructed, I will analyse the implications of this vulnerability and provide a geometric explanation for the existence of such perturbations via an analysis of the curvature of the decision boundaries.
- Speaker: Alhussein Fawzi; UCLA, DeepMind
- Tuesday 30 January 2018, 14:00–15:00
- Venue: Centre for Mathematical Sciences, MR4.
- Series: Mathematics and Machine Learning; organiser: Frank Kelly.